CAPE, a machine-learning model using thousands of chest X-ray images and data points, helps clinicians organise right level of care and monitoring based on risk score.
Amidst the pandemic, a multidisciplinary team from Changi General Hospital (CGH) and the Integrated Health Information System (IHiS) have developed a new artificial intelligence (AI) tool that can predict if an admitted patient will develop mild or severe pneumonia within 30 days based on his or her chest X-ray (CXR) image.
Called the Community Acquired Pneumonia and Covid-19 AI Predictive Engine (CAPE), this technology is especially helpful in identifying and prioritising the allocation of treatment resources for those who may need critical care support, an especially crucial concern during a pandemic.
“The conception of using AI to triage and predict the severity of pneumonia directly arose as a result of the [Covid-19] pandemic. Anticipating the severity of COVID-19 infections in patients was also one of the research areas identified by the World Health Organisation to tackle the disease,” said Dr Charlene Liew, Project Lead, Deputy Chief Medical Informatics Officer, CGH, and Director of Innovation, SingHealth Radiological Sciences Academic Clinical Programme (RADSC ACP).
Pneumonia is a main cause of deterioration in Covid-19 patients and is also a leading cause of death worldwide. The severity of pneumonia correlates to the degree of lung abnormality detected in a CXR. Recognising the potential in using AI to predict the progression of pneumonia, CGH’s Respiratory and Critical Care Medicine and Radiology teams partnered the IHiS Health Insights team to develop CAPE.
“Driven by the clinical care needs and resource demands of the pandemic, the CGH and IHiS teams saw the potential of AI to combat the critical needs of Covid-19,” said Professor Ng Wai Hoe, Chief Executive Officer of CGH.
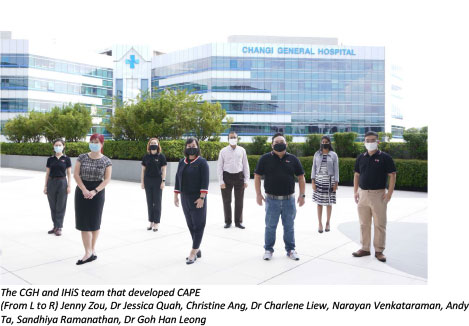
How it works
CAPE was trained to predict a patient’s pneumonia risk score through deep machine learning of more than 3,000 CRX images and 200,000 data points, including lab results and clinical history. When a patient is admitted into care, CAPE will instantaneously generate a score to indicate if the patient has low-risk pneumonia with an anticipated short hospital stay; the risk of mortality; or the risk of needing critical care support.
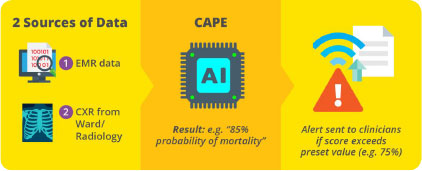
Conventionally, pneumonia assessment tools include analysing radiology images or electronic medical records data. CAPE is able to harness both sets of data to generate a risk score.
“We foresee CAPE to have a wide ranging impact upon the decision making process of organising the appropriate level of monitoring and care when they are admitted for pneumonia,” said Dr Jessica Quah, Consultant of Respiratory & Critical Care Medicine at CGH. In other words, clinicians can receive early warning for possible deterioration and prescribe appropriate interim measures to improve outcomes. These interim measures include the implementation of sepsis bundles such as antibiotics, fluid administration, and stabilisation of blood pressure, as well as early access to critical care support.
The technology currently has an accuracy rate of 80 per cent, comparable to traditional tools such as the Pneumonia Severity Index (PSI) and CURB-65 pneumonia severity risk calculator, which are scored manually.
Behind the ease and simplicity of CAPE, however, was the team’s tireless effort and the belief that “nothing is impossible”, according to Dr Liew.
“As with any deep learning model project, the strength lies in the volume of data used to train and test the model. The data preparation for model development was laborious due to the volume of data required,” said Narayan Venkataraman, Assistant Director of Data Management & Informatics at CGH.
Despite the hours needed for curating, validating, data mining and ensuring adherence to information security protocol, the team completed the development of CAPE in two months, a feat that was brought about by the urgency of tackling Covid-19.
Bruce Liang, CEO of IHiS said, “The accelerated launch and quick refinements were possible with an agile delivery approach and excellent partnership between the IHiS tech and CGH clinical teams.”
Dr Liew agreed, saying, “This was a multidisciplinary, complex project with many simultaneous moving parts. Like an orchestra, each player had to perform with outstanding dedication to their domain of expertise. It was daunting but an exciting journey of discovery at the same time.”
Prof Ng added, “CAPE shows the value of interdisciplinary collaboration and that research and innovation can occur even in difficult times to provide practical solutions to improve patient care.”
In recognition of its successful deployment, an academic paper written on CAPE was accepted as poster paper in “Knowledge Discovery and Data Mining” (KDD), a leading publication in data mining and analysis. The paper was also presented at the KDD conference in August 2020.
Next steps
Work is now underway to integrate even more clinical data from Singapore General Hospital and Sengkang General hospital and other SingHealth institutions so as to improve CAPE’s accuracy.
For wide-reaching impact, the team is planning to engage collaborators globally by hosting CAPE as a freeware on a research platform. The hope is that in time, CAPE can be used internationally.
Collaborators interested to work with CGH on CAPE can contact the Centre for Innovation at innovation@cgh.com.sg or Centre for Healthcare Assistive & Robotics Technology (CHART) at chart@cgh.com.sg